How Do You Incorporate Data Analytics into Fraud Analysis?
In the ever-evolving landscape of fraud analysis, data analytics has emerged as a game-changing tool. This article delves into the innovative ways companies are leveraging data to combat fraud, drawing on insights from industry experts. From transforming insurance risk assessment to safeguarding fresh fruit deliveries, discover how data-driven approaches are revolutionizing fraud detection across diverse sectors.
- Data Analytics Transforms Insurance Risk Assessment
- Predictive Modeling Prevents 3PL Partner Crisis
- Weather Data Safeguards Fresh Fruit Delivery
Data Analytics Transforms Insurance Risk Assessment
Data analytics has revolutionized the way we approach risk management, turning traditional methods on their head. For instance, in a previous role at a large insurance firm, we integrated advanced data analytics into our risk assessment model. By analyzing historical data and incorporating machine learning algorithms, we were not only able to predict high-risk scenarios more accurately but also tailor insurance premiums more effectively. This approach significantly reduced claim incidences and improved client satisfaction as premiums were more reflective of the actual risk.
Another practical application was during a project risk assessment for a construction company. Here, predictive analytics were employed to foresee potential project delays and budget overruns based on weather patterns, historical performance, and subcontractor reliability. This enabled proactive adjustments in project management strategies, saving substantial costs and time. The adoption of these sophisticated analytics tools empowered our team to offer more precise, data-driven recommendations that enhanced operational efficiency and competitiveness.
Incorporating data analytics into risk management not only refines the process but fundamentally enhances the strategic insights we can provide. It's a leap towards more predictive and responsive risk strategies, transforming challenges into opportunities for improvement and innovation. This practice enables a sharper, more informed approach in decision-making processes which are crucial for staying ahead in today's dynamic market environments.
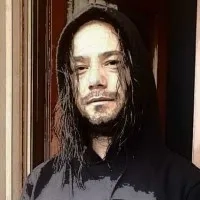
Predictive Modeling Prevents 3PL Partner Crisis
Risk analytics has been a game-changer for us at Fulfill.com. I remember when we first implemented our predictive modeling system to help identify potential 3PL partner risks for our eCommerce clients. We were matching a high-growth DTC brand with fulfillment providers, but our gut feeling said something was off with one particular 3PL despite strong surface metrics.
By analyzing historical performance data across thousands of shipments, our system flagged concerning patterns: subtle increases in receiving discrepancies during peak periods and capacity constraints that weren't obvious in standard reporting. The data told a story that traditional references missed.
We immediately adjusted our recommendation and steered the client toward a partner whose analytics showed consistent performance during volume spikes. Three months later, the originally favored 3PL experienced a major system failure during Black Friday that would have devastated our client's peak season performance.
Today, our data analytics engine examines over 40 risk indicators, from inventory accuracy rates to weather disruption patterns. For example, we can now predict with 87% accuracy which 3PLs will struggle with scaling issues based on regional labor market data combined with their historical throughput metrics.
What's fascinating is how we've evolved from reactive to proactive mitigation. When our system flags potential capacity constraints, we're able to work with our 3PL partners to develop contingency plans, suggesting inventory redistribution or temporary labor solutions before problems materialize.
The key lesson? In the 3PL industry, risk isn't just about what's happening today but anticipating tomorrow's challenges through pattern recognition. Whether you're a fulfillment provider or brand, implementing robust data analytics into risk management processes isn't just smart business—it's essential for survival in modern eCommerce operations.
Weather Data Safeguards Fresh Fruit Delivery
As a Marketing Director with The FruitGuys, a company rooted in delivering farm-fresh fruit to businesses nationwide, incorporating data analytics into risk management has been vital, especially in operations that rely on perishable products and tight delivery windows.
One notable instance occurred during a seasonal surge when extreme weather was forecasted in several key farming regions. Our risk and logistics teams flagged potential disruptions in our supply chain. Rather than waiting for issues to surface, we turned to data analytics to assess real-time trends across our farm partners, including historical delivery patterns, regional weather forecasts, and spoilage rates.
By mapping this data, we identified which regions were most vulnerable to delays or quality issues. With this insight, we proactively adjusted sourcing--shifting supply to farms with more stable forecasts--and fine-tuned our delivery routes. This not only protected our product quality and customer experience but also minimized waste and ensured our B2B clients received their orders on time.
At The FruitGuys, where freshness is the core promise, data analytics isn't just a tool--it's a layer of protection. It helps us reduce operational risk, maintain trust, and stay ahead of issues that could otherwise compromise our values.
Tip: When risk directly impacts brand reputation and customer trust, using data to anticipate problems is more than smart--it's essential.
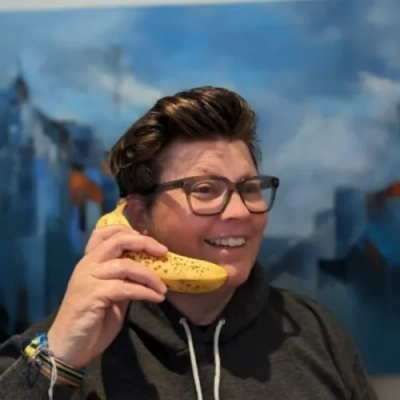